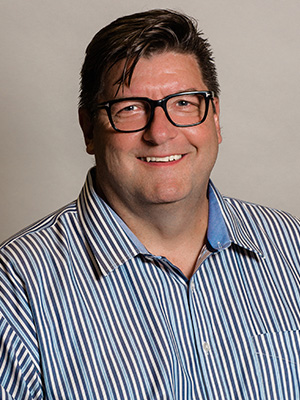
Artificial Intelligence: An Interview with Gary Saarenvirta, CEO, Daisy Intelligence Corporation
Gary Saarenvirta is the CEO of Daisy Intelligence Corporation, an award-winning artificial intelligence company specializing in retail merchandising solutions and insurance fraud detection.
Businesses are “drowning in data but starving for insight”, as the saying goes, and that problem is about to get worse. Marketers are facing a rising tide of digital interactions. Somewhere inside that massive pool of data are the answers to questions marketers haven’t even thought to ask – yet are essential to creating a better customer experience.
Relief is on the way in the form of artificial intelligence. The capability to skip right to the answers without even forming the questions will be the salvation of marketers. With AI, the analytical load shifts to machine learning algorithms. The first wave of commercial AI solutions has already made landfall. AI is being used today to improve audience targeting for programmatic media buying; make dynamic content and product recommendations; and drive demand-level pricing. Most of the major marketing automation vendors have already integrated AI capabilities into their platforms (like Salesforce’s Einstein). Companies also have the option of outsourcing the analytical work to software-as-a-service platform providers who will help them benefit from the technology immediately.
One of those SaaS providers is Toronto-based Daisy Intelligence founded by CEO Gary Saarenvirta. The company, which specializes in retail merchandising solutions and insurance fraud detection, was awarded the first-place prize at the 2018 Elevate AI Pitch competition. The AI platform developed by Daisy Intelligence can determine the best product price points; adjust the promotional mix to minimize cannibalization; identify optimal store locations and layout, and much more, saving merchandisers from needing to figure it out themselves. All the retailer needs to do is turn over as much transaction history as possible, and let the platform perform its magic.
AI is very much like magic because no one can ever say how it arrives at the answers it comes up with. Yet it offers clear advantages over traditional approaches to data mining and analysis, both in speed and precision, as Gary Saarenvirta explains.
Note: This interview has been edited and abridged for clarity and conciseness.
Stephen Shaw (SS): How did you come up with the “Daisy” name?
Gary Saarenvirta (GS): It was inspired by the first song ever sung by a computer back in 1961: Daisy Bell. And of course, that song was famously sung by HAL in “2001: A Space Odyssey.”
SS: It’s a perfect name for an AI company. What was the genesis of your business?
GS: My goal has always been to use math and science to make companies operate smarter. Take a typical retailer with hundreds of locations, a hundred thousand products, millions of customers – it’s hard to figure out what’s going on no matter how many analysts you have. Our vision was to build an autonomous decision-making system using artificial intelligence. That was 15 years ago.
SS: You’re one of the pioneers in the data mining business. In your career arc, what have been the key markers in the adoption of data analytics as a practice?
GS: Starting in the late 1990s, relational databases were starting to come on strong, resulting in these massive data warehouses. The arrival of parallel computing gave us a big step up in computing power, so you could actually use more sophisticated data mining tools to explore all of the data in these warehouses. More recently there has been a surge of interest in neural networks and that brought even greater attention to data mining and advanced analytics amongst banking and insurance companies, telcos, the biggest retailers – anyone with lots of data.
SS: I remember the analytical software market was very immature in those days.
GS: Yeah. Data mining technology has always been aimed at a technical user with a mathematical or statistics degree. Which is why a gap still exists today between data analytics and business decision making. It’s the main reason why analytics hasn’t become a strategic practice yet.
SS: In fact, half of companies report that they’re still struggling to create actionable insights. Why is that?
GS: The output of a predictive model is a numerical label or a text label: what do you do with that? There’s no decision-making process wrapped around that label. If the label says two instead of one, what do you do?
SS: Most marketers aren’t very data oriented. Is the problem a lack of data fluency?
GS: Yeah. The direct marketing world were the earliest adopters of advanced analytics. Companies like Reader’s Digest and American Express were pioneers using predictive modelling for direct mail. Today you see it being used for programmatic advertising. But what’s missing is a tie back to the P&L. At Air Miles – fantastic company, it was a formative place for me – I remember every PowerPoint report declaring, “This is a 100% ROI … a 500% ROI …. a 200% lift over random,” and that was all wonderful, but then the client’s P&L wouldn’t move. You saw all of this awesomeness from a statistical perspective, but it wasn’t translating into business results.
SS: You mean data mining is more of a tactical tool as opposed to strategic?
GS: Yeah, just think about the retail business. Maybe you did a shopper marketing campaign to sell Coke, and you sold twice as much Coke this week as last week. But then you don’t take into account the fact that Pepsi sales went down, juice sales went down. You don’t measure cannibalization. You don’t measure the forward buying: people who bought two cases this week because they were on sale, but don’t buy their usual case the following week. Marketers don’t bother to measure all of the ripple effects because that gets too complicated. I tell my customers If I don’t move the P&L, then fire me because there’s no point doing analytics if you’re not moving the P&L.
SS: Given the shortage of analytical talent, the argument can certainly be made that outsourcing is a more sensible option.
GS: I believe so. Imagine you’re a retailer and you hire an analytical person: what’s that person’s career path? I think analytics should all be outsourced. Even the big banks with their analytical centers of excellence, great, but the bank doesn’t have an analytics vision. The bank has a bank vision. The real analytical innovation can only come from outside the bank.
SS: I know that Toronto is viewed as an AI hotbed. Does that mean there’s a larger pool of people to draw upon here or is finding the right talent a real issue?
GS: We haven’t had a challenge finding technical people. Engineers and computational scientists are the people we go after. AI is a system and so you need engineering thinking. You can teach an engineer more math, but it’s hard to teach physics thinking or control theory to a computer scientist. So we’re collaborating with the University of Toronto and we’ve done hackathons with their engineering department.
SS: When you’re knocking on doors, are you talking to the CIO or the CMO? Who do you have to convince of the merits of AI?
GS: In retail, we want to talk to the CMO and the head of merchandising. Our goal in retail is to double the net income of retailers. We want to turn a 1% industry into a 6% industry. The people who care about that are in the C-suite. Our users are the retail operators, the merchandisers, the category managers, and the marketers who use our technology on a day-to-day basis. In insurance and banking, same thing, we go after the C-suite, the claims people, the risk people.
SS: In my own experience dealing with retailers, they’re notorious laggards when it comes to embracing innovation. Is that changing because of pressure from Amazon?
GS: Well, one of our longer-term client relationships has been Walmart and they’re technology innovators. It’s been a great relationship and we’ve been helping each other out. We have retail clients in four different geographies today, so it’s very interesting to see their needs coalescing. Technology is a key driver on the roadmap for all of them. Everyone’s really looking at technology as a key enabler and a survival mechanism.
SS: Not only do retailers have to deal with the omnichannel shopper, they’ve also got to deal with a sudden deluge of interaction data. They’re accustomed to looking at SKU-level data to make merchandising decisions, not customer-level data.
GS: That is a real challenge. One of the reasons I chose retail was because of the technical complexity of making use of all that data. Something like 50% of world GDP is retail. So if we can move the needle in retail, we can move the world.
SS: What phase is AI at? Is it still in a hype phase? An early adoption phase? Even a honeymoon phase?
GS: I think it’s still in the hype phase. Let me explain. If you only analyze historical data, that’s called statistical analysis. You learn from history only. You have to have labeled training examples to train your algorithm, whether that’s linear regression invented in 1805 or deep learning, which has become popular in the last 5 years. That’s all mathematical labeling. You create a label, a numerical number – this is a dog, that’s a cat. It’s just a label. It’s a mathematical process and it can only learn new things at the speed you collect new data.
SS: When you talk about deep learning, are you referring to neural nets?
GS: Neural nets or support vector machines or support vector regression, all of those things – it’s really all historical data learning. You can only learn a new mathematical pattern when you collect new data. If statistical analysis was a panacea, it would have had a greater impact long ago given the proliferation of statistical analysis tools. The hype is a whole new generation going, “Wow, this predictive modeling stuff is really powerful.”
SS: You mean it’s not a crystal ball.
GS: Right. What we do is different – it’s called reinforcement learning. Just imagine you’re building a self-driving car. You’d design that car using a computer simulation model where you can do a million hours of driving in one hour. There is no label training. You need no historical data to train the car – turn left, turn right, push the gas, brake: I call that reinforcement learning. Now, think about retail promotion. I have to pick 500 products to promote out of 100,000. But you can’t treat Coke and cheese and bread as three independent things. They’re all related. It’s the marketing mix that drives the result. In predictive analytics, I have to create a label for each mix. The combinatorial math tells me I could never come up with enough labels. So predictive analytics can’t work in that scenario. You have to use reinforcement learning. We can tell the retailer: here’s what you should promote, here’s the price, here’s the inventory allocation. Product price, placement and volume – those are the inputs, just like brake, gas, pedal, steering. That’s what we call autonomous decision making. That’s the big difference between AI and statistical analysis.
SS: What’s the business case you make to the merchandiser sitting across the table from you when you talk about this?
GS: We deliver decisions. If you execute the decisions, we’re going to grow your revenue by 3% to 5% or more and in a 1% net margin industry, we just doubled your profit. And we can actually deliver.
SS: And you do that by optimizing price and shelf allocation and promotions?
GS: And product selection. There are a lot of companies doing price optimization and forecasting, but I haven’t seen anybody help decide which products to pick. An example would be a product like ground beef. Consumers see ground beef promoted, they go, “Oh, I’m going to make an Italian dinner”.
SS: Now they have to buy associated products.
GS: So you buy pasta, tomato sauce, produce. If you’re making hamburgers, you buy hamburger buns and condiments. But because I bought ground beef to make hamburgers, I might not buy hot dogs. And then there’s forward buying where you’re stealing from the future. Plus the spatial geography. I’m not going to go out of my way to get a 10-cent discount on carrots. But if you’re giving gold bars away for free, I’ll drive across the world to get it. All the common-sense things that retailers know: lower prices equal more sales, front page of the flyer is better than back page. All these common-sense rules we’ve assembled into a theory of retail like the laws of physics.
SS: So that sounds phenomenal, obviously, but I’m struggling a little bit with what merchandisers might do differently using your model.
GS: Let’s use a household promotional flyer as an example. In the dairy category, I need to decide which products I’m promoting. Well, Daisy says, “Put milk on the front page, cheese and yogurt on the inside page and here’s the price you should charge.” So we tell the merchant, “Here’s what you should do.” In promotional planning there’s way too many data points for analysts to look at. One very large retailer was looking to do 90 billion product forecasts 3 times a day. 90 billion. They had several million products and thousands of locations. They wanted to know three times a day what the demand for those product combinations would be at the store level. These problems are beyond human capability to compute. That’s the class of problems AI should focus on.
SS: AI has a liberating effect. If you can relieve marketers of the complexity, they can actually focus on other things where human judgment and intuition are more important.
GS: Absolutely. I like the word “liberating” because that’s one of our founding principles: by making the world smarter and more efficient we can eliminate human drudgery.
SS: Defeat data tyranny.
GS: Yeah, absolutely. That’s what our belief is. There’s loads of things people do that machines are terrible at, you know? There’s all this hype about “singularity”. It’s completely overblown. In the creative world, human beings excel at ambiguity. That’s the marriage between the two sides.
SS: Where else can AI have an immediate business impact?
GS: Anywhere involving large volumes of data where highly complex decisions need to be made. In insurance, we’re doing fraud detection and predictive underwriting, for example, where you might get a million claims coming in every day, needing to decide what’s fraudulent. Another example is screening bank transactions for money laundering or speeding up mortgage approvals which might happen thousands of times a day. Again, just moving the needle 1% or 2% can have a significant financial impact.
SS: Is AI going to govern the real-time interaction experience of customers?
GS: We’re analyzing 100% of the transactions and interactions of our retail clients across all channels: in-store, online, mobile, you name it. Using that data we’re able to help our retailers give their customers what they want, which is having the products available that they’re interested in, at prices they find compelling, and having the stock there when they go to buy the product.
SS: Do you also use AI to identify micro-segments within the base that you might start thinking about differently?
GS: Yeah, I’m a believer in segments and so in our retail simulation model, we decompose the business into customer segments and store segments. You can have hundreds or thousands of segments in a million-customer database.
SS: Is there a risk with AI that it will actually create greater distance between marketers and analytics because those decisions are now being handed off to a machine?
GS: You know, I think that’s a good point. It could happen. I think customers are always asking, “So what’s inside the black box?” We try to be as transparent as possible. But it’s so complex. We might do a hundred trillion computations to come up with an answer. There needs to be some element of trust. But it’s not like the machines will take over all analysis and humans will do none.
SS: So you’re saying there’s still a role for predictive analytics and the sister disciplines?
GS: Yeah, certain problems. A customer acquisition challenge. A targeting challenge. Those are perfect predictive modeling opportunities. So there are certain problems where predictive analytics will play a role and certain problems where reinforcement learning is better.
SS: What do you see as the future of AI over the next number of years? How do you see it evolving and maturing?
GS: I think you’re going to hear more about systems and control theory being applied to AI systems. How do we control these systems, how do we make them fault tolerant? If I order a million tons of bananas, whoops, that should have been 100,000, it could really hurt the business. The system has to be fault tolerant.
SS: What’s the on-ramp for AI in a company that hasn’t been down this path before?
GS: Start with a big problem. Then look at business processes where people struggle, where there’s lots of data, super complicated trade-offs, dependent on old rule-based systems. Figure out how the math is going to help. If you’re going to create a predictive model, think about what you’re going to do with the answer. And there’s still a lot of data management issues that haven’t been addressed. You need to manage your master data, your product hierarchies, your historical promotions. All of that drives the quality of AI.
SS: Data quality is still an obstacle?
GS: It still is. To me, it’s the proof point that nobody is really doing any strategic analytics because we haven’t solved that problem yet. With every retailer we work with, the first thing we do is tons of data management work. For example, you have all these different UPC codes – like all the different flavours of yogurt – which should be considered as one group. That data entity doesn’t exist in the vast majority of retailers. Large retailers only very, very recently, in the last year or two, started to actually think about those things. Which is proof that analytics hasn’t even scratched the surface of possibilities.
SS: As a long-time data mining advocate, it must be gratifying to see all of this interest in AI.
GS: I’ve been at this for 25 years and finally my vision is starting to gain traction. At times I thought I was out of my mind. So, yeah, it’s very exciting to have people buy into the vision.
Stephen Shaw is the chief strategy officer of Kenna, a marketing solutions provider specializing in customer experience management. He is also the host of a monthly podcast called Customer First Thinking. Stephen can be reached via e-mail at sshaw@kenna.ca.